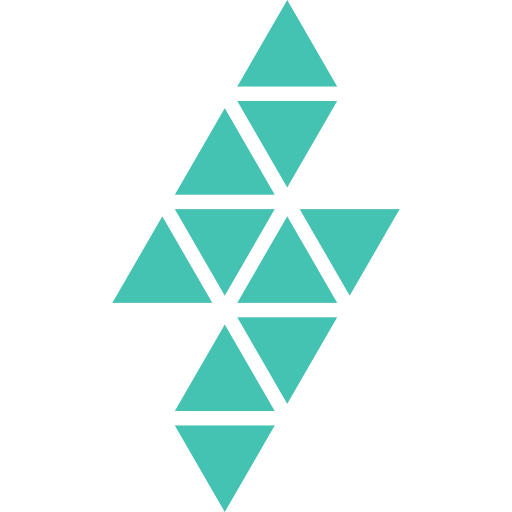
Snapdragon Tech Blog
Musings of a systems administrator and open source developer
Update 2021: See here for another great collection of different email spoofs by Kaspersky.
Recently I noticed a rise in spam emails with spoofed From-headers. This is unusual because almost all mail servers will require users to log in before sending emails. Below a typical example, which was already flagged as spam.As you can see, the sender- and recipient addresses are the same in Apple Mail. The content implies that the user’s mailbox was hacked.
I have blogged about how great Borg is to back up servers and your Macbook while on the go. There just wasn’t a good hosting service to stash your backup repos that took full advantage of all Borg features. Issues I saw with existing services, like Hetzner’s Storagebox and rsync.net:
Only one single user. If a machine gets compromised they can access all your backups. No support for append-only mode.
Updates:
Oct 2018: there is now a more detailed guide available for macOS. Sept 2018: there is now a hosting solution for Borg repos. See this post When I recently switched my Macbook, I got very frustrated with Time Machine. I had used it for occasional local backups of my home folder and was planning to move my data from the new to the old machine.
Unfortunately, the Migration Assistant failed to even find my Time Machine drive and I ended up simply rsyncing everything from the Time Machine backup to a new user folder.
This page summarizes the settings needed to configure clients to access your email, contacts and calendars.
Email 🔗Webmail: https://mail.snapdragon.cc
For checking email in your browser, changing password or setting filters.
Apple Configuration Profile: https://profile.snapdragon.cc
Automatically installs required settings for iOS and macOS devices. (Recommended)
Microsoft Outlook Setup: If autodiscover is enabled for your domain, just enter your email and password to set up the account. Be sure to use the full, rather than simplified dialog.
Step 1: Install certificate files 🔗Open and install all the certificate files you received. Before installing your private certificate, you will need to enter the installation password.
Step 2: Restart Apple Mail 🔗After successfully installing all certificate files, restart Apple Mail
Step 3: Sending encrypted email 🔗After restarting Apple Mail you will see two new icons when composing an email.
The lock icon denotes an encrypted email. This message is encrypted with the recipient’s public key and can only decrypted using his private key.
Step 1: Install Maildroid and Crypto Plugin 🔗Maildroid works with IMAP mail servers and has actively-supported S/MIME encryption capabilities. Install the app via the app store or these links:
https://play.google.com/store/apps/details?id=com.maildroid
https://play.google.com/store/apps/details?id=com.flipdog.crypto.plugin
There is also an add-free paid version called Maildroid Pro.
Step 2: Private Certificate Installation 🔗After installing Maildroid and setting up your email account, copy your private certificate file (ending in .p12) to the device. This can be done via USB-connection or by sending and saving the file as attachment.
Step 1: Install private certificate 🔗You should have received your private certificate via email in an encrypted .p12 file. This file is password-protected to keep it save until it arrives on your device. It’s always recommended to distribute the .p12 file and corresponding password via separate channels.
After opening the attachment, iOS will offer to install the certificate as identity profile. Choose install.
If you happened to have a passcode on your device, iOS will ask you for your passcode and then the certificate password.
Step 1: Import certificates 🔗In this step we will import your personal certificate. Please open Thunderbird and navigate to
Tools > Options > Advanced > View Certificates
Under Your certificates, you can see your own certificates. People shows other user’s public certificates.
To import your own certificate click Import and select the .p12 file you received by email.
After entering the certificate password you received by email, you should see your Name and expiration information.
Step 1: Install certificates 🔗Double click the certificate file (or multiple files) you received by email in Outlook or Windows Explorer. Just keep the default options of each step and click next until the certificate is installed. At some point you need to enter the certificate password, you received by SMS.
Step 2: Set up certificate in Outlook 🔗File > Options > Trust Center > Trust Center Settings > Email Security
Updates are important. While not as great as on Linux, you can use different package managers to manage all installed software and easily keep applications updated. Here the command I use. Requires homebrew, homebrew-cask-upgrade and mas.
function update-all { # update cli homebrew brew update brew upgrade brew prune brew cleanup # Homebrew cask (via https://github.com/buo/homebrew-cask-upgrade) brew cu -a -y --cleanup brew cleanup --force -s && rm -rf $(brew --cache) # Node npm update -g # Apple App store mas upgrade softwareupdate --install --all }